Technology has revolutionized the way how businesses understand and engage with their customers.
It is not just in terms of indefinite predictions but also with concrete data-backed insights.
That’s essentially what predictive analytics does. It’s the art and science of using historical data to predict future outcomes.
The global data marketplace platform market generated a revenue of USD 1,201.9 million in 2023.
Source: Grand View Research
Moreover, the market is expected to reach USD 5,739.2 million by 2030.
To capitalize on this growth, businesses increasingly turn to a specialized Marketing Analytics & Reporting Agency that offers expert insights and tailored strategies.
The blog post will cover what is predictive analytics in marketing and how to forecast and improve future campaigns.
Understanding Predictive Analytics
Predictive analytics in marketing harnesses various data analytics techniques to forecast future events and consumer behaviors.
By analyzing past and current data, marketers can create predictive models that help refine marketing strategies, ensuring they are more aligned with the anticipated needs and behaviors of their target audience.
Source: Grand View Research
This branch of analytics involves a robust integration of marketing analytics, customer behavior data, and advanced statistical methods to forecast the outcomes of marketing campaigns.
The core of predictive analytics lies in its ability to turn raw data into actionable insights. For instance, data analytics and reporting play a pivotal role in capturing and interpreting customer interactions across multiple channels.
How Predictive Analytics Works
The mechanism of predictive analytics in marketing is rooted deeply in data analytics techniques that sift through vast datasets to identify patterns and trends that human analysts might miss. The process typically follows several key steps:
- Data Collection: This foundational step involves gathering data from various sources such as social media, transaction records, customer surveys, and website interactions. The goal is to amass a comprehensive dataset that reflects the diverse aspects of customer behavior and business operations.
- Data Preparation: The collected data often comes in raw and unstructured forms that need cleaning and organizing. This step ensures that the data is accurate and consistent, removing anomalies and errors that could skew the predictive models.
- Data Analysis: At this stage, data analytics and reporting tools come into play, processing the cleaned data using sophisticated algorithms and statistical methods. Analysts use techniques like regression analysis, clustering, and decision trees to explore the data and identify significant correlations and patterns.
- Model Building: The insights gleaned from data analysis are used to create predictive models. These models are essentially algorithms that, when applied to new data, can predict outcomes such as customer responses to a particular marketing strategy or the probable success of a marketing campaign.
- Validation and Testing: Predictive models aren’t deployed immediately after creation. They must first be validated and tested against a separate set of data to ensure their accuracy and reliability. This step is crucial for refining the models and adjusting parameters to improve prediction accuracy.
- Deployment: Once validated, the predictive models are integrated into marketing strategies. They begin influencing decisions, from personalized marketing campaigns targeting specific customer segments based on predicted behavior to adjustments in budget allocation across different marketing channels.
- Monitoring and Updating: Predictive models are not static; they require ongoing monitoring and updating to remain effective. Changes in customer behavior, market conditions, or the business environment can all influence the model’s accuracy. Regular updates ensure the models adapt to these changes, maintaining their effectiveness over time.
Predictive analytics empowers marketers to not just react to customer behaviors but to anticipate them.
By understanding potential future actions of customers, companies can craft proactive marketing strategies that align closely with expected behaviors, enhancing customer engagement and optimizing marketing effectiveness.
Benefits of Predictive Analytics in Marketing
Predictive analytics in marketing offers a significant competitive advantage by leveraging digital marketing analytics to anticipate customer needs, optimize marketing strategies, and ultimately increase profitability.
As businesses seek to understand what is marketing analytics, they increasingly turn to predictive models that use complex algorithms, including machine learning techniques and neural networks, to forecast future events.
Enhanced Customer Insights
The first and perhaps most profound benefit of predictive analytics in marketing is the ability to gain deep customer insights.
By analyzing historical data through sophisticated marketing analytics software, companies can predict future customer behaviors and preferences with a high degree of accuracy.
This process involves examining a vast array of data points, from purchasing history and online browsing habits to social media interactions and geographic information.
Using machine learning techniques, predictive analytics tools can process this data to identify patterns and trends that are not immediately apparent.
Additionally, the global Machine Learning (ML) market size was valued at USD 19.20 billion in 2022.
Source: Fortune Business Insights
For instance, neural networks may simulate human thought processes in pattern recognition, providing insights into customer behaviors that help marketers tailor their offerings more precisely.
This level of insight enables businesses to create highly personalized marketing strategies that resonate with individual customers, enhancing engagement and loyalty.
Improved Campaign Efficiency
Another significant advantage of predictive analytics is its ability to enhance the efficiency of marketing campaigns.
Predictive analytics marketing leverages accurate trend prediction to allocate resources more effectively, ensuring that marketing dollars are spent on the right channels and toward the right audiences.
Marketing analytics software equipped with predictive capabilities can analyze past campaign performances and predict the outcomes of future campaigns under different scenarios.
This informed decision-making process helps marketers optimize their budget allocation, focusing on strategies that offer the highest return on investment (ROI).
Source: Return on investment (ROI) of advertising campaigns, Statista
Increased Revenue Opportunities
Finally, predictive analytics drives revenue growth by identifying lucrative upsell and cross-sell opportunities.
Through detailed customer data analysis, predictive models can suggest which products a customer is likely to buy next, based on their purchase history and browsing behaviors.
This insight allows companies to present customers with personalized recommendations at critical points in the customer journey, such as during online checkout or through personalized email marketing.
Implementing Predictive Analytics
Implementing predictive analytics in marketing is a transformative step for any organization, driving more informed decisions and refining marketing efforts through data-driven insights.
This complex process involves several key stages, from the initial setup of predictive analytics systems to the integration of insights into everyday marketing strategies.
Starting with Predictive Analytics
The first step in implementing predictive analytics in marketing involves laying the foundational elements necessary for success.
Businesses must assess their current data infrastructure to ensure it can handle the demands of predictive analytics marketing. This means evaluating existing marketing analytics software and determining if it can integrate new data sources that predictive analytics will require.
Assess Data Quality
Begin by auditing your current data for quality and completeness. Predictive analytics requires high-quality, granular data to produce accurate predictions.
Issues like missing data, data silos, or outdated information can significantly impair the accuracy of your predictive models.
Upgrade Technology
Many organizations may find that their current marketing analytics software lacks the necessary capabilities for advanced predictive modeling.
Investing in specialized predictive marketing analytics tools that can handle large datasets and complex algorithms is crucial. These tools should offer features specific to predictive analytics in marketing, such as customer churn prediction or content marketing analytics.
Skill Development
Ensure your team has the right skills to manage and interpret predictive analytics. This may involve training current staff or hiring new talent specialized in B2B marketing analytics, predictive modeling, and data science.
Set Clear Objectives
Define what you aim to achieve with predictive analytics. Common goals include improving customer engagement, optimizing marketing spend, reducing churn, and increasing conversion rates.
Objectives should be specific, measurable, achievable, relevant, and time-bound (SMART).
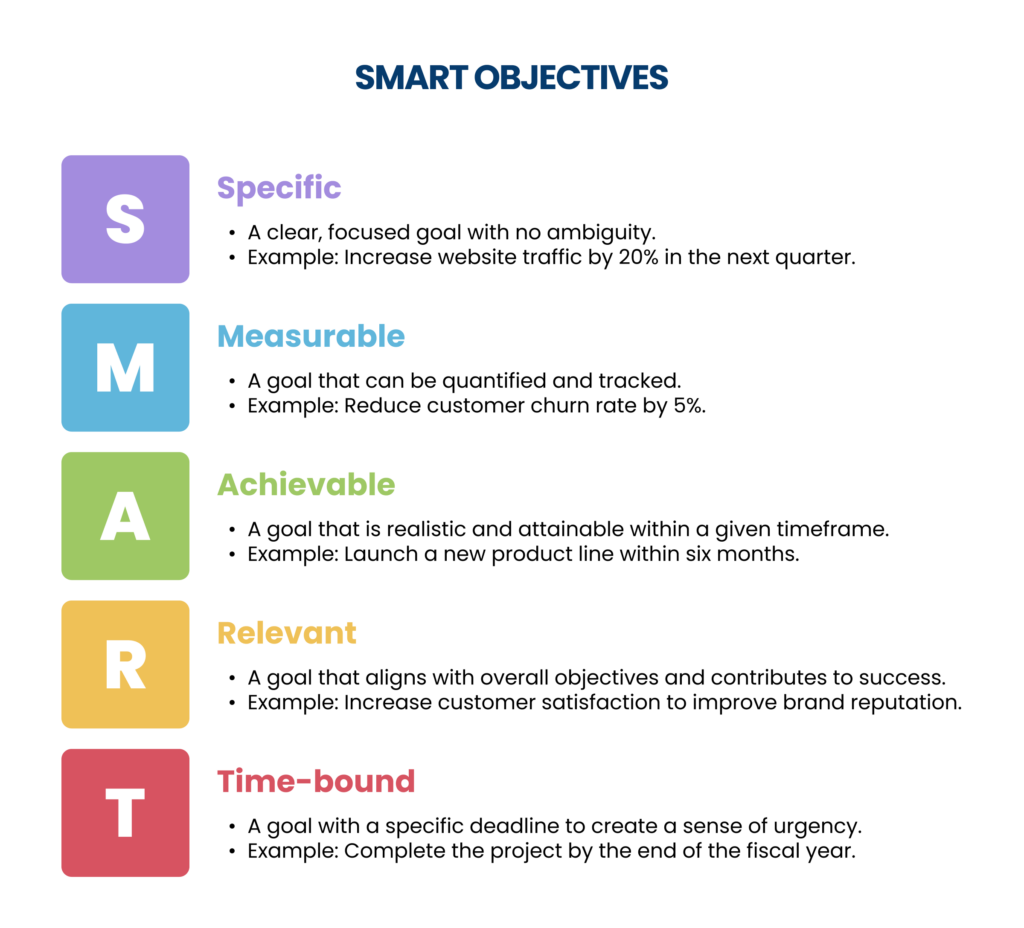
Building Predictive Models
Once the groundwork is laid, the next step is to develop predictive models that will generate the insights needed to drive decision-making.
Predictive analytics models are constructed by selecting algorithms that best fit the business problem and training them with historical data to forecast outcomes.
Data Preparation
Gather and prepare the data required for modeling. This involves cleaning the data, dealing with missing values, and possibly creating new variables that can help in making more accurate predictions.
Selecting the Right Algorithms
Choose algorithms that best suit the type of data and the specific prediction tasks, whether it’s predicting customer behaviors, forecasting sales trends, or identifying potential high-value customers.
Types of Models
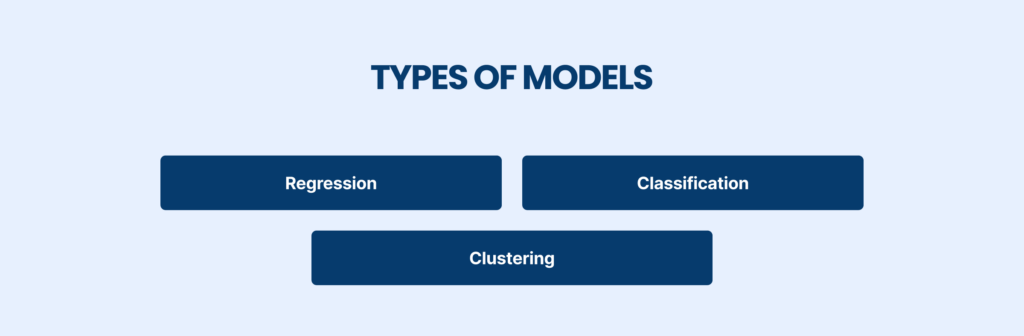
- Regression Models
These are used when the prediction involves a continuous outcome. For example, regression models could predict the exact amount of money a customer will spend in the next month based on their past shopping behaviors.
- Classification Models
These models are applied when the outcome is a categorical variable. For instance, classification can be used to predict whether a customer will churn or not, turning this into a binary outcome: yes or no.
- Clustering Models
Clustering is useful for segmenting the customer base into distinct groups without prior knowledge of those groups. This can be particularly useful in targeted marketing campaigns, as it allows for the customization of marketing messages based on segmented customer characteristics.
Integrating Predictive Insights
After developing predictive models, the next challenge is integrating these insights into marketing strategies effectively.
Embedding Predictive Insights into Marketing Strategies
This involves taking the predictions from your models and using them to inform marketing decisions.
For example, insights from email marketing analytics can inform the timing, content, and audience of your email campaigns to increase engagement and conversion rates.
Embedding Predictive Insights into Marketing Strategies
The integration process often requires close collaboration between data scientists and marketing teams to translate predictive outputs into actionable marketing strategies.
It might involve setting up automated systems where predictive insights directly adjust marketing efforts in real-time.
- Real-time Decision Making
Utilize predictive insights to make real-time adjustments to marketing campaigns. For instance, if predictive analytics indicate an emerging trend in customer preferences, marketers can immediately shift their focus to capitalize on this trend, potentially gaining a first-mover advantage.
- Continuous Learning and Improvement
As predictive models are deployed, it’s vital to continuously monitor their performance and adjust as necessary. This iterative process helps refine the models and ensures they remain accurate as market conditions change.
Future of Predictive Analytics in Marketing
Predictive analytics in marketing is not a static field; it continuously evolves with technological advancements and changing market dynamics.
As businesses strive to stay competitive, understanding the future trends in predictive analytics can provide valuable insights into how marketing strategies might be shaped in the coming years.
Emerging Trends and Innovations
The landscape of predictive analytics in marketing is witnessing rapid changes, driven by both technological advancements and shifts in consumer behavior.
One of the most significant trends is the increasing ability to predict future customer behavior with greater accuracy and detail.
This capability is transforming how businesses approach everything from product development to personalized marketing campaigns.
Customer Segmentation
Advanced predictive analytics tools are enhancing customer segmentation by utilizing deeper data insights to classify customers into more precise and meaningful groups based on predicted behaviors, preferences, and value to the company.
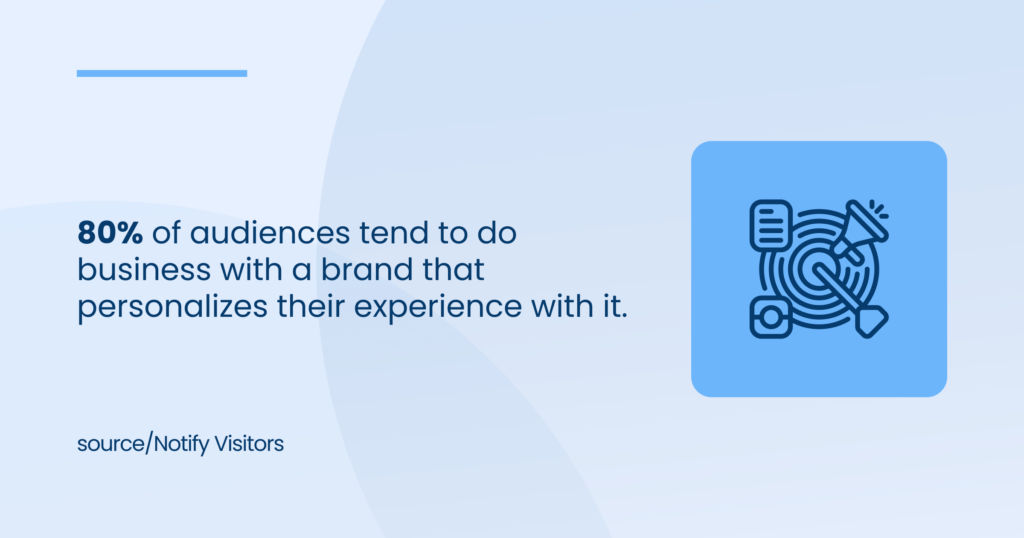
This refined segmentation helps marketers tailor their efforts more effectively, enhancing customer satisfaction and loyalty.
Predictive Customer Journeys
Marketers are increasingly using predictive analytics to map out future customer journeys. By predicting the paths customers are likely to take, businesses can optimize every touchpoint along the way, from personalized ads and tailored email marketing campaigns to customized shopping experiences.
Real-time Predictive Analytics
The future of predictive analytics also points towards real-time data processing. This allows businesses to adjust their marketing strategies instantly based on dynamic customer interactions and behaviors.
Real-time analytics enable marketers to deliver highly relevant offers and content at the perfect moment, thereby significantly increasing the chances of conversion.
Machine Learning Advancements
Machine learning is at the heart of predictive analytics, and its advancements are crucial in driving the field forward.
As machine learning algorithms become more sophisticated, they enhance the predictive capabilities of analytics tools, leading to more accurate forecasts of market trends and customer behaviors.
Deep Learning
Deep learning models, which are a more complex subset of machine learning, are being increasingly utilized for their ability to analyze large sets of unstructured data such as images, text, and voice.
marketing, this means improved understanding of customer sentiments and intentions, which can predict customer behavior with unprecedented precision.
Automated Machine Learning (AutoML)
AutoML is becoming more popular, as it allows marketers with limited data science expertise to deploy machine learning models.
Source: Fortune Business Insights
This democratization of technology means predictive analytics can be used more widely across different levels of an organization, enhancing decision-making at all levels.
Enhanced Personalization
Machine learning advancements are enabling ultra-personalized marketing strategies by predicting not just if a customer will engage but how they will engage with different content types, channels, and messaging.
This level of personalization is crucial in a world where consumers are increasingly expecting experiences tailored to their unique preferences and needs.
Integration with Other Technologies (IoT, Blockchain)
The integration of predictive analytics with other technologies like the Internet of Things (IoT) and blockchain is setting the stage for innovative applications in marketing.
IoT and Predictive Analytics
IoT devices generate vast amounts of data from connected devices such as smartphones, wearables, and home appliances.
By applying predictive analytics to this data, marketers can gain more detailed insights into customer habits and environments, leading to highly contextualized marketing messages.
For example, a smart fridge could inform grocery brands when a consumer is running low on a product, triggering timely and relevant ads or offers.
Blockchain for Enhanced Data Security
Blockchain technology is starting to play a role in predictive analytics by providing a secure environment for data sharing. In marketing, blockchain can enable the secure exchange of customer data across platforms without compromising privacy.
This capability is particularly important as concerns about data privacy continue to grow, and regulations become more stringent.
Cross-Technology Synergies
The synergy between blockchain, IoT, and predictive analytics is creating new opportunities for marketers to engage with customers. For instance, blockchain can authenticate the data from IoT devices, ensuring its accuracy before it’s used in predictive models.
This integration ensures that the insights derived are both reliable and robust, paving the way for more innovative and effective marketing strategies.
Partner with [A] Growth Agency for Your Marketing Analytics and Reporting Strategies
As technology continues to evolve, the importance of marketing analytics and reporting will only grow.
Businesses rely increasingly on data-driven insights to understand past performances and forecast future trends and consumer behaviors.
[A] Growth Agency will be your reliable support in the process. We are a team where data-driven strategies meet creative experiments.
We believe in building lasting partnerships for lasting success. With us, you will gain a partner committed to understanding your unique challenges and opportunities.
Don’t settle for less.
Go ahead and Get Your Free Marketing Plan